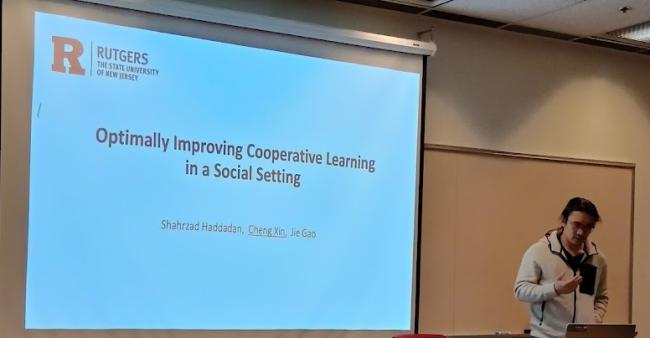
Cheng Xin and Kevin Lu, students advised by Jie Gao, delivered flash talks inspired by their ACTION-related research at the DIMACS Workshop on Spreading on Social Networks – Theory and Applications, held at Rutgers University from October 21, 2024 - October 23, 2024. The event was co-sponsored by the Center for BioComplexity, High Meadows Environmental Institute, Princeton University and The Santa Fe Institute.
Abstracts for both presentations follow:
Optimally Improving Cooperative Learning in a Social Setting
Cheng Xin
We consider a cooperative learning scenario where a collection of networked agents with individually owned classifiers dynamically update their predictions, for the same classification task, through communication or observations of each other’s predictions. Clearly if highly influential vertices use erroneous classifiers, there will be a negative effect on the accuracy of all the agents in the network. We ask the following question: how can we optimally fix the prediction of a few classifiers so as maximize the overall accuracy in the entire network. To this end we consider an aggregate and an egalitarian objective function. We show a polynomial time algorithm for optimizing the aggregate objective function and show that optimizing the egalitarian objective function is NP-hard. Furthermore, we develop approximation algorithms for the egalitarian improvement. The performance of all of our algorithms is guaranteed by mathematical analysis and backed by experiments on synthetic and real data.
Enabling Asymptotic Truth Learning in a Social Network
Kevin Lu
Consider a network of agents that all want to guess the correct value of some ground truth state. In a sequential order, each agent makes its decision using a single private signal which has a constant probability of error, as well as observations of actions from its network neighbors earlier in the order. We are interested in enabling network-wide asymptotic truth learning – that in a network of n agents, almost all agents make a correct prediction with probability approaching one as n goes to infinity. In this paper we study both random orderings and carefully crafted decision orders with respect to the graph topology as well as sufficient or necessary conditions for a graph to support such a good ordering. We first show that on a sparse graph of average constant degree with a random ordering asymptotic truth learning does not happen. We then show a rather modest sufficient condition to enable asymptotic truth learning. With the help of this condition we characterize graphs generated from the Erdös Rényi model and preferential attachment model. In an Erdös Rényi graph, unless the graph is super sparse (with O(n) edges) or super dense (nearly a complete graph), there exists a decision ordering that supports asymptotic truth learning. Similarly, any preferential attachment network with a constant number of edges per node can achieve asymptotic truth learning under a carefully designed ordering but not under either a random ordering nor the arrival order. We also evaluated a variant of the decision ordering on different network topologies and demonstrated clear effectiveness in improving truth learning over random orderings.