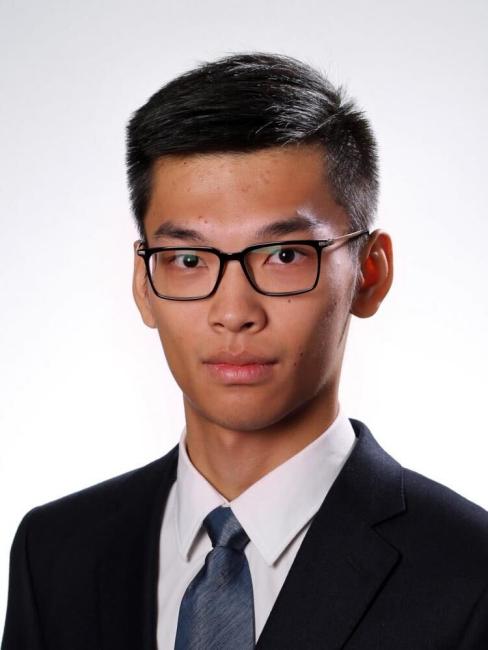
Chong Xiang
Thursday, 28 March 2024
11 am - 12 pm PT
Join via Zoom
Abstract
Existing defenses for AI models are being broken by adaptive attacks all the time. One major reason is that most defenses do not provide convincing explanations or formal proof of any model robustness they have intended to achieve.
In this talk, I will present our research efforts toward overcoming this challenge. Specifically, I will take the local corruption attack (a.k.a. adversarial patch attack in computer vision) as a case study to demonstrate how we can design algorithms with certifiable/provable robustness. The certifiable robustness aims to provide a provable guarantee that robustness claimed by the defender (for a certain threat model) will not be compromised by any attacker within the same threat model, including white-box adaptive attackers with full knowledge of the defense setting.
I will cover three defense algorithms: PatchGuard, PatchCleanser, and PatchCURE. Notably, this series of defenses can achieve strong certifiable robustness for large datasets and models, only at a minimal cost of model utility (e.g., 1% clean accuracy drop for ImageNet-1k). In addition, I will discuss the potential generalization of these algorithms to different tasks, modalities, models, and attacks, as well as alternative pathways toward provable and explainable AI security.
Bio
Chong is a fifth-year PhD student at Princeton University where he is advised by Prof. Prateek Mittal. His research studies the security and privacy vulnerabilities of AI models and systems. He has received the Award for Excellence and Yan Huo *94 Graduate Fellowship at Princeton University.
Hosted by ACTION Student Advisory Council (Shinan Liu, Head)