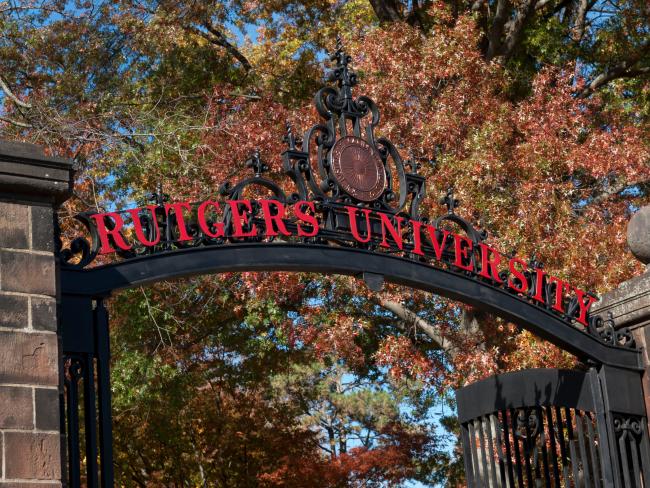
At Rutgers University, four REU students worked on topics related to ACTION in Summer 2024. Two of the students, Filip Uradnık and Julia Krizanova, hailed from Charles University and were funded by Czech republic. The other two students, Amanda Wang and Rhett Olson were from Princeton University and the University of Minnesota, respectively.
Under the supervision of Dr. Jie Gao, the Rutgers interns worked on a project titled, "Hardness and robustness to adversarial players in social learning." Truth learning, i.e., inferring the ground truth of an unknown signal from private noisy observations and peer predictions through a network, is a very challenging task. There are two types of challenges. On one hand, the prediction accuracy cannot be improved if there are not enough opportunities to aggregate noisy observations. On the other hand, an excessive amount of information flow can lead to a phenomenon called herding or information cascade where agents drop their own signals and conform to the prediction of the 'crowd.' Here we examine the question of whether the topology of a given network supports truth learning or not. We show that this decision problem is in fact NP-hard by using a reduction from a classical 3-SAT problem. In addition, we also examine network topologies that not only support truth learning but also do so despite the existence of adversarial agents.
In Summer 2025, the ACTION Institute anticipates expanding the summer internship program even further. Please reach out if you are interested in participating. Find more information on our Education and Outreach page.